- همه نشریات
- نشریات علمی سازمان تحقیقات، آموزش و ترویچ کشاورزی
- مجلات ترویجی سازمان تحقیقات، آموزش و ترویج کشاورزی
- سایر نشریات سازمان تحقیقات، آموزش و ترویج کشاورزی
- نشریات علمی انجمن ها
- علوم آب و خاک
- علوم اقتصاد و ترویج کشاورزی
- علوم جنگل, مرتع و آبخیزداری
- علوم دامی و شیلات
- علوم زراعی و باغی
- علوم گیاهپزشکی
- علوم مهندسی و مکانیزاسیون کشاورزی
- نشریات با زبان انگلیسی
- نشریات با زبان فارسی
- نشریات دانشگاه آزاد اسلامی
- نشریات علمی
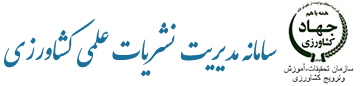